Optimization of Multi – object Radar Resolution
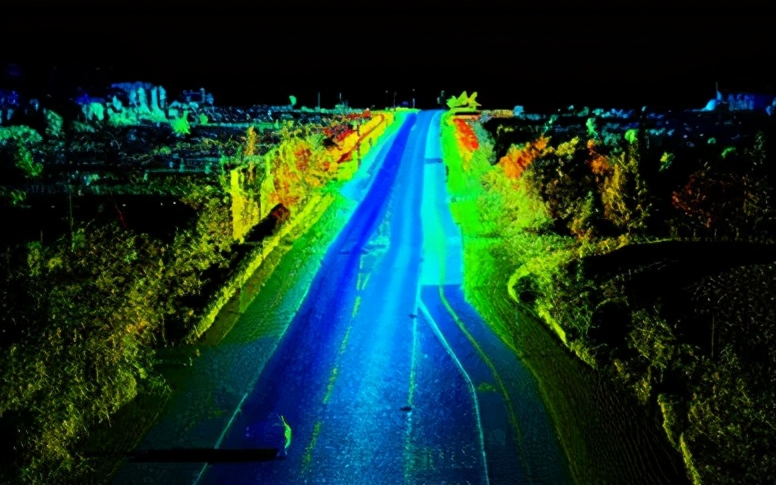
In the powerful drive of the global intelligence wave, multi – object radar systems have become indispensable core sensing devices in many key fields. According to the authoritative forecast of MarketsandMarkets, during the period from 2023 to 2030, the annual compound growth rate of the radar market will reach 8.2%. This data fully demonstrates the broad development prospects of radar technology. In the field of autonomous driving, multi – object radars are key sensors to ensure the safe driving of vehicles and realize intelligent decision – making. At the national defense security level, they shoulder the important task of monitoring the dynamics of airspace and sea area and safeguarding national security. In the construction of smart cities, multi – object radars assist in traffic management, environmental monitoring, etc., improving the operational efficiency of cities and the quality of residents’ lives.
However, traditional radars have insufficient resolution when dealing with complex and dense object scenarios. Taking urban traffic radars as an example, according to the data of IEEE in 2023, during peak traffic hours, the object loss rate is as high as 30%. This phenomenon not only seriously affects the performance of radars but also may trigger a series of safety hazards and management problems. Therefore, it is urgent to deeply study the technology of optimizing the resolution of multi – object radars. This article will systematically analyze the technical paths for optimizing the resolution of multi – object radars from the three dimensions of physical principles, algorithm innovation, and engineering practice. Combining with the cases of industry leaders such as NASA, Huawei, and Tesla, it will deeply reveal the future development trends of the industry.
1. Resolution Limits: Physical Laws and Engineering Challenges
1.1 Physical Boundaries of Radar Resolution
The resolution of a radar is mainly limited by two classic formulas, which fundamentally determine the accuracy of the radar in distance and angle measurement.
The range resolution formula is , where represents the speed of light, a constant value of approximately , and is the signal bandwidth, which reflects the frequency range of the radar – transmitted signal. From this formula, it can be seen that the larger the signal bandwidth , the smaller the range resolution , and the stronger the radar’s ability to distinguish objects at different distances.
The angular resolution formula is , where represents the wavelength, which is related to the frequency of the radar – transmitted signal; is the number of array elements, that is, the number of independent radiation units in the radar antenna array; and is the element spacing. In this formula, the shorter the wavelength , the larger the number of array elements , or the larger the element spacing , the smaller the angular resolution , and the higher the radar’s ability to distinguish objects at different angles.
A more intuitive understanding of the resolution differences of different types of radars can be obtained through the comparison of typical systems:
Radar Type |
Bandwidth (GHz) |
Range Resolution (cm) |
Angular Resolution (°) |
Vehicle – mounted Millimeter – wave Radar |
4 – 7 |
3.75 – 6.25 |
1.5 – 5 |
Military Early – warning Radar |
0.5 – 2 |
75 – 300 |
0.1 – 0.5 |
Synthetic Aperture Satellite |
0.1 – 0.5 |
300 – 1500 |
0.01 – 0.1 |
When the actual object spacing is less than the range resolution , or the angular difference between objects is less than the angular resolution , problems will occur. At this time, the echo signals received by the radar will be aliased in the time – domain and spatial – domain. It’s like the “voices” of different objects overlapping, making it difficult to distinguish. In this case, the traditional matched – filter algorithm will fail, and the signal – to – noise ratio loss will exceed 15 dB. The signal – to – noise ratio is an important indicator for measuring the quality of radar – received signals. A significant decrease in the signal – to – noise ratio means that the useful information in the radar – received signals is submerged by noise, making it impossible to accurately identify and distinguish objects.
1.2 Multi – object Signal Model and Interference Mechanism
The mathematical model of the radar – received signal can be expressed by the formula . In this formula, represents the reflection coefficient of the – th object, which reflects the object’s ability to reflect radar signals. Objects with different materials, shapes, and sizes have different reflection coefficients; represents the time delay, where is the distance between the radar and the – th object. The time delay is proportional to the object distance. The farther the distance, the longer the time delay; is the Doppler shift, where is the radial velocity of the object and is the center frequency of the radar – transmitted signal. The Doppler shift is related to the object speed. The faster the speed, the larger the frequency shift; and represents noise, which is an inevitable interference factor in the process of radar receiving signals.
When the object spacing satisfies or , cross – term interference will occur in the time – frequency domain. This interference will cause the radar to generate incorrect object information during signal processing, that is, the so – called “Ghost Objects”. These “Ghost Objects” do not actually exist but can mislead the radar’s judgment and bring great trouble to object detection and tracking.
2. Technological Breakthroughs: Multi – dimensional Innovations from Algorithms to Hardware
2.1 Revolution of Super – resolution Algorithms
2.1.1 Compressed Sensing
The traditional Nyquist sampling theorem requires that the sampling frequency be at least twice the highest frequency of the signal to accurately reconstruct the signal. However, compressed sensing technology breaks this limitation. It uses the sparsity of signals to achieve super – resolution reconstruction. In actual radar application scenarios, the distribution of objects in space is often sparse, that is, most of the space has no objects. Compressed sensing is based on this characteristic. Through a small amount of sampling data, it can accurately restore the position information of objects.
Relevant experiments conducted by MIT Lincoln Laboratory have fully verified the effectiveness of compressed sensing technology. The experimental results show that the object position can still be restored at a sampling rate of only 10%, and the mean – square error is less than . This means that even when the sampling data is severely insufficient, the compressed sensing algorithm can still determine the object position with high accuracy, providing a new idea for improving radar resolution.
Its core formula is . The meaning of this formula is to solve for that minimizes under the constraint condition . Here, is the radar – received signal, is the measurement matrix, and is the object signal to be restored. By solving this optimization problem, a high – resolution object signal can be restored from a small amount of measurement data .
2.1.2 Driven by Deep Learning
The rise of deep – learning technology has brought new opportunities for radar signal processing. RadarNet developed by the Fraunhofer Institute in Germany adopts the U – Net architecture. The U – Net is a neural network architecture widely used in the field of image processing. It has a unique encoder – decoder structure and can effectively extract feature information in images. RadarNet applies the U – Net architecture to radar signal processing and realizes the separation and identification of multiple objects through end – to – end training. Experimental data shows that RadarNet has increased the multi – object separation accuracy to 94.7%, while the traditional method is only 78.2%. This significant improvement fully demonstrates the powerful capabilities of deep learning in radar signal processing.
In 2023, Huawei released the RadarFormer model, which innovatively applied the Transformer to the field of radar signal processing. The Transformer is a neural network architecture based on the self – attention mechanism. It can extract and fuse features from data in different dimensions. RadarFormer uses the self – attention mechanism to achieve three – dimensional decoupling across range – Doppler – angle. In dense traffic scenarios, the false alarm rate is reduced by 62%. This achievement enables radars to more accurately detect and track objects in complex traffic scenarios, providing strong support for the development of fields such as autonomous driving.
2.2 MIMO Radar and Virtual Aperture Technology
The technical principle of MIMO (Multiple – Input Multiple – Output) radar is to transmit orthogonal waveforms. In this way, the MIMO radar can construct virtual channels (where is the number of transmitting array elements and is the number of receiving array elements). Compared with traditional radars, the increase in virtual channels is equivalent to expanding the radar aperture, thus effectively improving the radar’s resolution. The resolution improvement factor is .
The Tesla HW4.0 radar adopts a 4D imaging MIMO architecture with an array scale of . This large – scale array design enables the radar to achieve an azimuth resolution of , a range resolution of 2 cm, and can simultaneously track 512 objects. In the autonomous driving scenario, such high resolution and powerful object – tracking capabilities enable vehicles to more accurately sense the surrounding environment, detect potential hazards in advance, and make more reasonable driving decisions.
The US military’s AN/SPY – 6 radar has achieved a major breakthrough in detection accuracy through digital sub – array – level MIMO technology, with a 30 – fold improvement compared to the previous – generation radar. This improvement enables the US military to more accurately detect and track objects in national defense tasks such as air defense and anti – missile, greatly enhancing national defense capabilities.
2.3 Photonic Radar and Quantum Enhancement
Photonic – assisted radars use optical frequency combs to generate ultra – wideband signals, and their bandwidth can exceed 10 GHz. The use of ultra – wideband signals can effectively improve the radar’s range resolution. The experimental system of Japan’s NICT achieved an angular resolution of in the 77 – GHz band, demonstrating the huge potential of photonic – assisted radars in improving resolution. The introduction of photonic technology can not only improve the performance of radars but also has the advantages of small size, light weight, and strong anti – electromagnetic interference ability, providing a new direction for the miniaturization and high – performance development of radars.
Quantum radars use the properties of quantum mechanics to improve radar performance. The quantum noise radar developed by China Electronics Technology Group Corporation No. 38 Research Institute uses entangled photon pairs to increase the signal – to – noise ratio by 20 dB. In a strong – interference environment, its detection probability is as high as 95%. Compared with traditional radars, quantum radars have obvious advantages in detecting weak objects and anti – interference. They can more accurately detect objects in complex electromagnetic environments, providing more reliable technical support for national defense security and other key fields.
3. Industry Implementation: The Leap from Laboratory to Commercialization
3.1 Intelligent Transportation: Millimeter – wave Radar Matrix
The Hangzhou City Brain Project has actively explored and practiced in the field of intelligent transportation and deployed a 128 – channel 60 – GHz radar array. This radar array adopts a distributed beam – forming algorithm, which can flexibly adjust the direction and shape of the radar beam according to different object distributions and environmental conditions, thereby improving the radar’s detection performance.
In terms of key indicators, the object capacity of this radar array reaches 500 vehicles per square kilometer, which means that the radar can simultaneously detect and track 500 vehicles in an area of one square kilometer, meeting the high requirements for vehicle monitoring in complex urban traffic scenarios; the positioning error is less than 10 cm, ensuring accurate measurement of vehicle positions; and the power consumption is less than 15 W per node, achieving low – power operation and reducing operating costs.
The technical highlights of this project include multi – radar cooperative SLAM (Simultaneous Localization and Mapping) technology. Through the collaborative work of multiple radars, a more accurate and complete urban traffic map can be constructed, providing a more reliable basis for vehicle positioning and navigation. At the same time, the dynamic parameter optimization technology based on federated learning can dynamically adjust the parameters of the radar according to real – time traffic data and environmental changes, improving the adaptability and performance of the radar.
3.2 National Defense Security: Multifunctional Phased – array Radar
The Lockheed Martin LRDR radar is an important equipment in the field of national defense security, operating in the S – band (2 – 4 GHz). The radar has more than 50,000 array elements, and its large number of array elements provides a hardware basis for its high performance.
In terms of performance parameters, the detection range of the LRDR radar exceeds 2,000 km, enabling effective monitoring of long – distance objects; and it can simultaneously track more than 1,000 objects, with strong multi – object tracking capabilities. To further improve the resolution, the radar adopts a combination of Space – Time Adaptive Processing (STAP) and machine – learning technologies. The STAP technology can adaptively process radar signals in the spatial and time domains to suppress clutter and interference; the machine – learning technology can continuously optimize the radar’s signal – processing algorithm based on a large amount of training data, improving the accuracy of object detection and recognition.
3.3 Environmental Monitoring: Space – borne SAR System
The BIOMASS satellite of the European Space Agency is equipped with a P – band synthetic aperture radar (435 MHz). The choice of the P – band enables the radar to have a strong vegetation – penetration ability and can penetrate vegetation more than 1 m thick.
In terms of application results, the satellite has achieved remarkable results in forest biomass mapping, with a mapping error of less than 20%, providing accurate data support for forest resource monitoring and ecological environment research; in glacier thickness monitoring, the accuracy reaches m, helping scientists to better understand the changes of glaciers and providing important basis for dealing with climate change.