Performance Optimization Strategies for Microwave Vehicle Detectors
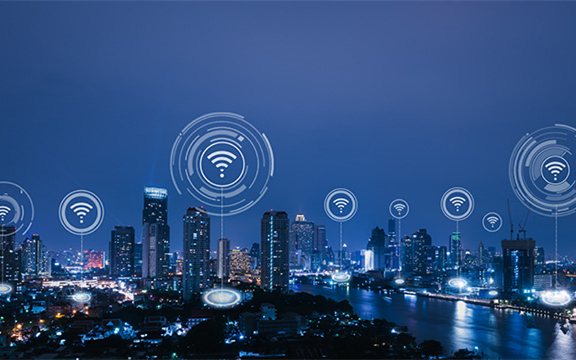
In the context of the booming development of intelligent transportation systems, microwave vehicle detectors (MVDs), as crucial core sensing devices in these systems, have become the focus of attention in the entire industry regarding performance optimization. Microwave vehicle detectors with excellent performance can accurately obtain various information about vehicles, providing key data support for traffic flow regulation, intelligent driving assistance, etc., thus ensuring the efficient and safe operation of the traffic system. This article will delve into multiple dimensions, including technology research and development, hardware design, algorithm upgrades, and system integration. By combining current industry trends and cutting – edge successful cases, it comprehensively and meticulously explores the performance optimization strategies for microwave vehicle detectors, aiming to provide valuable references for industry practitioners.
Ⅰ. Technology Research and Development: Intelligence and Multimodal Fusion
1. Integration of Intelligent Sensing and Deep Learning Algorithms
The core function of a microwave vehicle detector is to accurately identify the position, speed, and vehicle type of vehicles using microwave signals. However, traditional detection algorithms have obvious shortcomings. In practical applications, they are highly susceptible to interference from environmental noise. For example, in urban areas, microwave signals reflected by surrounding buildings and electromagnetic noise generated by other electronic devices can all affect the detection results, ultimately leading to a high false detection rate.
In recent years, deep – learning technology has emerged as a powerful force and has played a significant role in improving the detection accuracy of microwave vehicle detectors. Take the convolutional neural network (CNN) as an example. It can process microwave detection signals more efficiently and intelligently. By constructing multiple convolutional and pooling layers, CNN can automatically extract key features from the signals, enabling real – time and accurate discrimination between vehicles and interfering objects. For instance, the features of trees in microwave signals are significantly different from those of vehicles, and CNN can identify them accurately. In rainy and snowy weather, although microwave signals are attenuated and scattered, CNN can still distinguish vehicle signals from complex signals, reducing the false alarm rate to less than 1%.
Some innovative manufacturers have further expanded the application scope of deep learning and developed license plate recognition models. When integrated with the microwave vehicle detection function, this model can not only classify vehicle types but also automatically count traffic flow. By recognizing license plates, the system can more accurately track the driving trajectories of vehicles, providing more detailed and precise data for traffic management departments and facilitating the formulation of more scientific and reasonable traffic management strategies.
2. Multimodal Sensing Technology Fusion
Although microwave detection technology is widely used in the field of vehicle detection, single – microwave detection has non – negligible limitations in the face of extreme weather or complex road conditions. For example, in heavy rain or thick fog, microwave signals are severely attenuated and scattered, resulting in a significant decrease in detection accuracy. In areas with dense traffic flow and complex road structures, microwave signals are prone to reflection and refraction, interfering with the detection results.
To address these issues, multimodal sensing technology fusion has emerged. By integrating multi – source data from radars, cameras, geomagnetic sensors, etc., a more robust detection system can be constructed. Take the “radar – vision integrated machine” as an example. It skillfully combines the speed – measuring ability of microwave radars with the visual recognition advantages of video cameras. In rainy and foggy weather, the microwave radar can use its penetration ability to roughly determine the position and speed of vehicles, while the camera can use its visual imaging function to identify details such as the vehicle’s shape, color, and license plate. Complementing each other, they can still maintain high – precision detection even in harsh weather conditions.
In addition, the application of multimodal data fusion is not limited to vehicle detection but can also be used for traffic incident warning. Through the comprehensive analysis of multi – source data from radars, cameras, etc., the system can promptly detect abnormal traffic incidents such as 逆行 and congestion. When it detects that the driving direction of vehicles in a certain lane is opposite to the normal direction, the system will quickly issue a 逆行 warning. When it detects that the vehicle density in a certain section is too high and the driving speed is significantly reduced, the system will determine it as congestion and promptly notify the traffic management department so that corresponding traffic – calming measures can be taken, greatly improving traffic management efficiency.
In terms of technological breakthroughs, the application of non – equidistant microstrip array antennas and long – baseline phase – comparison angle – measuring technology is of milestone significance. In the past, long – distance vehicle angle measurement has always been a difficult problem in microwave vehicle detection. Due to the long signal transmission distance and many interference factors, the angle – measurement error was large, resulting in a high lane – division error rate. The application of these two technologies has effectively solved this problem. The non – equidistant microstrip array antenna can optimize the radiation pattern of the antenna, improving signal gain and directivity. The long – baseline phase – comparison angle – measuring technology can achieve high – precision vehicle angle measurement by accurately measuring the phase difference of signals received by different antennas. Working together, they have reduced the lane – division error rate by 40%, greatly enhancing the performance of microwave vehicle detectors.
Ⅱ. Hardware Design: High Precision and Low Power Consumption in Parallel
1. Chip Performance and Signal Processing Optimization
The core hardware of a microwave vehicle detector is undoubtedly the radar chip, which directly determines the detection performance of the device. Currently, the mainstream solution adopts the 24GHz high – frequency band (bandwidth 245MHz) and linear frequency – modulated continuous wave (LFMCW) technology. This technology combination has many advantages, and the most significant one is that it can achieve a ranging accuracy of 0.1 – meter level. In practical applications, whether it is a fast – moving vehicle on the highway or a slow – moving vehicle on urban roads, the distance between the vehicle and the detector can be accurately measured.
However, the industry is constantly evolving, and the pace of innovation never stops. Phased – array radar technology has become an important innovation direction for the future hardware development of microwave vehicle detectors. Phased – array radar technology can achieve dynamic beam adjustment by controlling the phase and amplitude of multiple antenna elements. This means that microwave vehicle detectors can flexibly change the direction of the detection beam according to actual needs, enabling synchronous detection of multiple lanes. At the same time, through real – time monitoring and analysis of vehicle movement trajectories, it can also predict vehicle driving trajectories, providing more forward – looking data support for traffic management and intelligent driving.
2. Low – Power Design and Sustainability
Considering that microwave vehicle detectors usually need to operate outdoors for a long time, the issues of energy supply and environmental protection cannot be ignored. To adapt to this application scenario, the device must balance energy consumption while ensuring performance.
Currently, some innovative solutions have achieved good results in practical applications. For example, the solar – power – supply scheme uses solar panels to convert solar energy into electrical energy, providing a stable energy supply for the device. In sunny areas, solar power can not only meet the daily operation requirements of the device but also store excess electricity for use at night or in bad weather conditions. In addition, the dynamic power management strategy is an effective means to reduce energy consumption. This strategy automatically adjusts the device’s power consumption according to the device’s working state and actual detection needs. When there are no vehicles in the detection area, the device can enter a low – power standby mode. When a vehicle enters the detection area, the device quickly switches to the normal working mode, thus realizing dynamic energy – consumption management. Compared with traditional power management methods, the power consumption can be reduced by 30%.
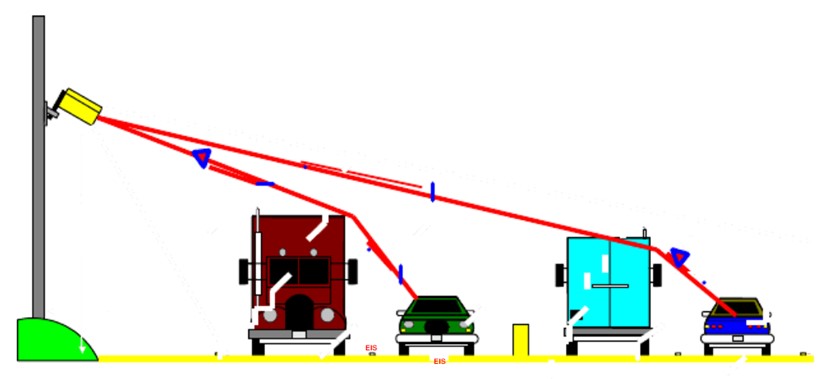
Ⅲ. Improvement of Anti – Interference Ability and Reliability
1. Environmental Interference Suppression Technology
Complex electromagnetic environments and harsh weather conditions are the main challenges faced by microwave vehicle detection. In urban areas, various electronic devices and communication base stations can generate electromagnetic interference, affecting the normal transmission and detection of microwave signals. In harsh weather conditions such as rain, snow, and hail, microwave signals are severely attenuated and scattered, leading to a decrease in detection accuracy.
To meet these challenges, a series of advanced environmental interference suppression technologies have emerged. The adaptive filtering algorithm is an important representative. It can automatically adjust the parameters of the filter according to changes in environmental noise, effectively suppressing interference signals. For example, when encountering electromagnetic interference, the adaptive filtering algorithm can quickly identify the frequency and characteristics of the interference signal and remove it from the detection signal by adjusting the filter coefficients, ensuring the accuracy of the detection results.
The Doppler compensation technology is mainly used to deal with changes in Doppler frequency shift caused by vehicle movement and weather factors. In rainy and snowy weather, the movement of raindrops or snowflakes also generates Doppler frequency shift, which is superimposed on the Doppler frequency shift of vehicles, affecting detection accuracy. The Doppler compensation technology eliminates the influence of these interference factors through accurate calculation and compensation of the Doppler frequency shift, increasing the detection stability of the device in rain, snow, hail, and other conditions by 50%.
In addition, some manufacturers have developed anti – metal interference algorithms for the problem of signal crosstalk in adjacent lanes. In actual traffic scenarios, most vehicles are made of metal materials. The metal components of vehicles in adjacent lanes can reflect and scatter microwave signals, resulting in signal crosstalk. The anti – metal interference algorithm effectively reduces the signal crosstalk in adjacent lanes through the analysis and processing of signal characteristics, further improving the detection accuracy.
2. Redundancy Design and Fault Self – Detection
To ensure the reliable operation of microwave vehicle detectors in various complex environments, redundancy design and fault self – detection technologies are crucial. The dual – radar beam system is a typical redundancy design scheme. It sets up two independent radar beam detection areas to achieve redundant detection of vehicles. When one beam fails, the other beam can still operate normally, maintaining the basic detection function of the device and ensuring the continuity and reliability of detection.
At the same time, the built – in self – detection module of the device can monitor the operating state of the device in real – time. The self – detection module regularly detects key components of the device, such as the radar chip, signal processing circuit, and communication module. Once a fault is detected, it immediately issues an alarm and quickly locates the fault point through the fault diagnosis algorithm. In sections with heavy traffic flow, rapid fault repair can reduce the loss of traffic data caused by device failures, ensuring the stable operation of the traffic management system.