The Innovation and Practical Application of Traffic Flow Prediction Technology
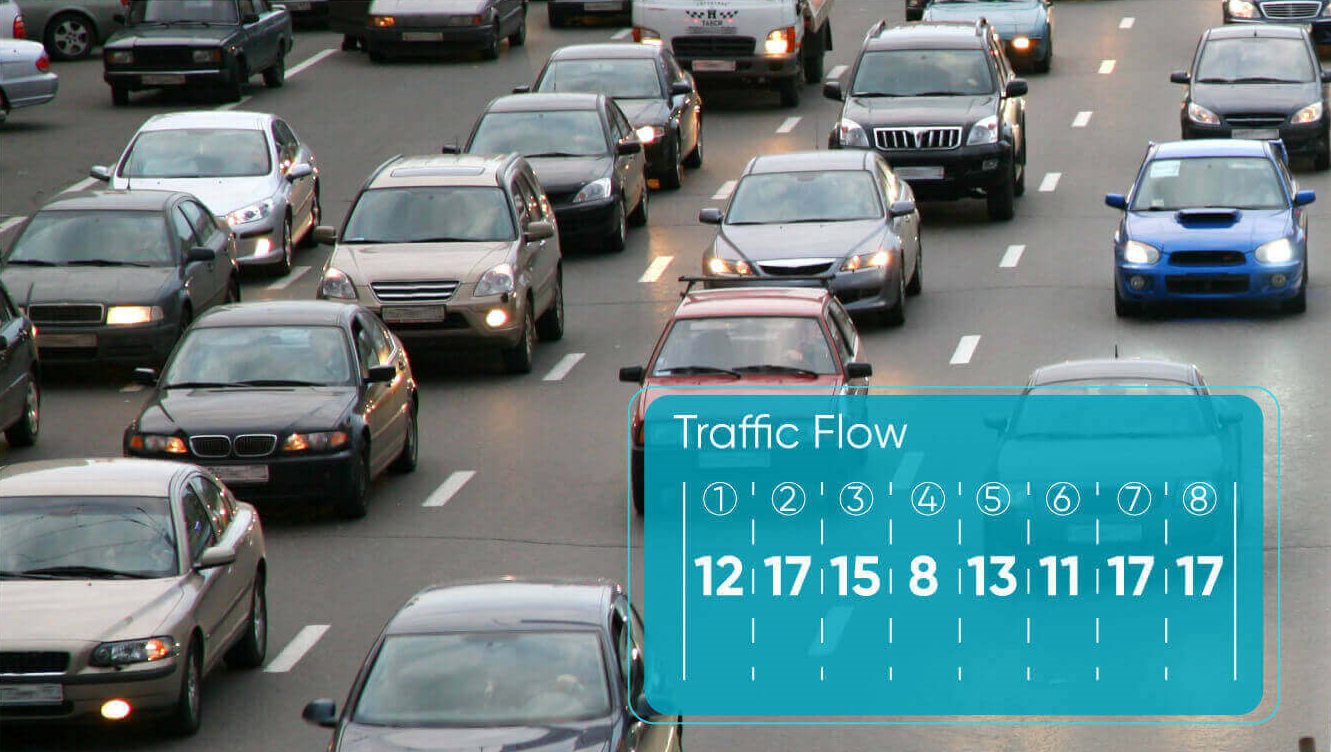
The research findings of “Traffic Flow Prediction and Big Data Analysis” released in March 2025 indicate that significant progress has been made in traffic flow prediction models constructed by integrating multi – source data, which can achieve a precise balance in short – term and long – term predictions. This breakthrough is of great significance for urban traffic management and planning, providing a more scientific and accurate basis for traffic decision – making. It is worth noting that the fusion model based on LightGBM + Transformer – GRU has performed exceptionally well in recent empirical studies. In terms of the R² index for evaluating the goodness of fit of the model, this fusion model reached 0.93, far exceeding traditional algorithms and fully demonstrating its excellent prediction performance.
1. Technological Innovation: Algorithmic Revolution and Data Integration
1.1 Breakthroughs in Deep Learning and Hybrid Models
The field of traffic flow prediction is undergoing a major paradigm shift from traditional statistical models to deep learning models. Traditional statistical models have many limitations when dealing with complex traffic data. Deep learning, with its powerful automatic feature learning ability and complex model construction ability, brings new development opportunities for traffic flow prediction. The latest research in 2025 fully confirms that organically combining the attention mechanism based on Transformer with the time – series modeling ability of GRU can effectively capture the correlations of traffic flow in the time and space dimensions.
In practical applications, the LightGBM + Transformer – GRU architecture shows unique advantages. Firstly, as a gradient – boosting decision tree algorithm, LightGBM can efficiently screen out the key features affecting traffic flow, such as weather conditions and accident rates. These features are crucial for accurately predicting traffic flow, because changes in weather may affect people’s travel intentions and modes, and traffic accidents directly reduce road capacity. Secondly, Transformer, using its self – attention mechanism, can deeply explore the spatial dependencies in traffic flow and accurately capture the mutual influence of traffic flow between different regions. Finally, GRU, with its strong modeling ability for time – series data, conducts time – series predictions on the screened and processed data. Experimental results on the Matlab platform show that compared with traditional models, this architecture reduces errors by 28%, significantly improving prediction accuracy.
Multimodal data fusion technology is also an important aspect of breakthroughs in deep learning and hybrid models. An empirical project carried out in São Paulo, Brazil, provides strong evidence for the effectiveness of this technology. This project fuses GPS trajectory data, mobile phone signaling data, and weather data, leveraging the complementarity of different types of data to comprehensively and accurately reflect traffic conditions. Among them, GPS trajectory data can accurately record the driving paths and speeds of vehicles, mobile phone signaling data can reflect the movement of people, and weather data takes into account the impact of external environmental factors on traffic. By fusing these data, the project’s prediction accuracy has increased by 41%, fully demonstrating the great potential of multimodal data fusion technology in improving the accuracy of traffic flow prediction.
In addition, edge computing optimization technology also plays an important role in traffic flow prediction. Deploying the CNN model on roadside edge devices and using the local computing power of edge devices can achieve real – time prediction of traffic flow. Experimental results show that this solution can achieve a real – time prediction response at the 50ms level, and the efficiency is 6 times higher than that of traditional cloud computing solutions. This optimization can not only obtain traffic flow information in a timely manner but also greatly reduce data transmission delays, providing more timely and accurate decision – making support for traffic management.
1.2 Innovation in Big Data Processing Paradigms
With the explosive growth of traffic data volume, the innovation of big data processing paradigms has become the key to the development of traffic flow prediction technology. In the construction of dynamic OD matrices, the city is divided into 95 regional units through the bus stop clustering algorithm. This division method can more delicately reflect the traffic demands of different urban areas. Moreover, the passenger flow matrix updated every 15 minutes can accurately and in real – time reflect the state of the urban traffic network, providing a timely and accurate data basis for traffic flow prediction.
Spatio – temporal feature engineering is an important part of the innovation of big data processing paradigms. An improved K – means algorithm is used to process the GPS data of 2,000 buses, constructing a 12 – dimensional feature vector that includes traffic connection strength, regional economic indicators, etc. These feature vectors comprehensively consider the time and space characteristics of the traffic system, as well as the impact of regional economic development on traffic flow, and can more comprehensively describe the changing laws of traffic flow, providing more representative input data for subsequent prediction models.
Outlier processing technology is crucial for ensuring the accuracy of traffic flow prediction. The outlier detection model based on the self – attention mechanism has achieved remarkable results in the Hangzhou pilot project, successfully identifying 98.7% of abnormal traffic events. This model can automatically learn the patterns and characteristics of normal traffic flow. Through the analysis of real – time data, it can timely detect abnormal situations that deviate from the normal patterns, such as traffic accidents and road construction, providing an important basis for traffic management departments to take emergency measures.
2. Practical Applications: The Intelligent Transformation of Urban Traffic
2.1 Applications in Traffic Management Scenarios
In 2024, the intelligent prediction system deployed by the Beijing Traffic Management Bureau effectively improved the traffic efficiency during the morning peak by 23% through a series of innovative measures. The dynamic signal control system played an important role. Based on the LSTM – based phase prediction model, this system can predict the changing trend of traffic flow 5 minutes in advance and adjust the signal timing plan accordingly. By reasonably allocating the green light time in different directions, it reduces the waiting time of vehicles and improves road capacity. Accident impact prediction is also an important function of this intelligent prediction system. The spatio – temporal diffusion model integrated with traffic accident data can accurately predict the congestion range based on information such as the location, time, and type of the accident. Experimental results show that the congestion range prediction accuracy of this model reaches 89%. The Beijing Traffic Management Bureau’s elastic departure system, which combines passenger flow prediction, adjusts the departure frequency of buses according to real – time passenger flow. The application of this system has reduced the standard deviation of the line load factor from 35% to 12%.
2.2 Innovations in Public Travel Services
The personalized prediction function introduced in the Shenzhen Traffic APP provides more convenient and efficient services for the public, reflecting three major technological breakthroughs. In terms of user profile fusion, the APP integrates the travel habit data of 5 million users. Through data analysis and mining technology, 32 types of travel mode tags are generated. These tags can accurately describe the travel preferences and habits of different users, providing personalized travel suggestions for users. Multi – objective path planning is another highlight of the Shenzhen Traffic APP. This function uses the Pareto – optimal algorithm considering time, cost, and comfort to provide users with multiple travel options. Every day, the average daily service decision – making of this function exceeds 2 million times, helping users find the most suitable travel path among many options and improving travel efficiency and satisfaction.
2.3 Decision – making Support for Urban Planning
Traffic flow prediction technology has played a key role in Shanghai’s 2035 traffic planning. In the evaluation of road network carrying capacity, the Monte Carlo simulation method is used to simulate and predict different traffic construction plans, analyzing their impacts on road network carrying capacity. By comparing the differences in the effects of different plans, it provides a scientific basis for urban planners to help them select the optimal construction plan to meet the future development needs of urban traffic. TOD development optimization is also an important application of traffic flow prediction technology in urban planning. The station passenger flow classification system combined with rail transit prediction can accurately predict the passenger flow of different stations. In emergency evacuation simulation, traffic flow prediction technology can simulate the traffic flow situation under extreme weather conditions such as heavy rain. Through the prediction and analysis of traffic flow, the error is controlled within 15%.
The traffic flow prediction technology has achieved remarkable results in both technological innovation and practical applications, providing strong support for the intelligent transformation of urban traffic. With the continuous development and improvement of technology, it is believed that traffic flow prediction technology will play an even more important role in future urban traffic management and planning.