Optimizing traffic flow models using traffic sensor data
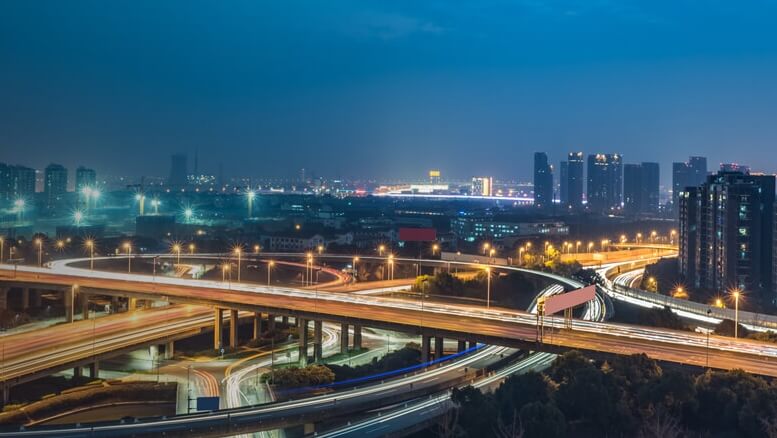
In modern cities, traffic congestion has become an important factor restricting urban development. With the development of intelligent transportation systems (ITS), traffic sensors, as the main source of real-time data, are crucial for optimizing traffic flow models. This article will provide a detailed introduction on how to use traffic sensor data to optimize traffic flow models and improve urban traffic efficiency.
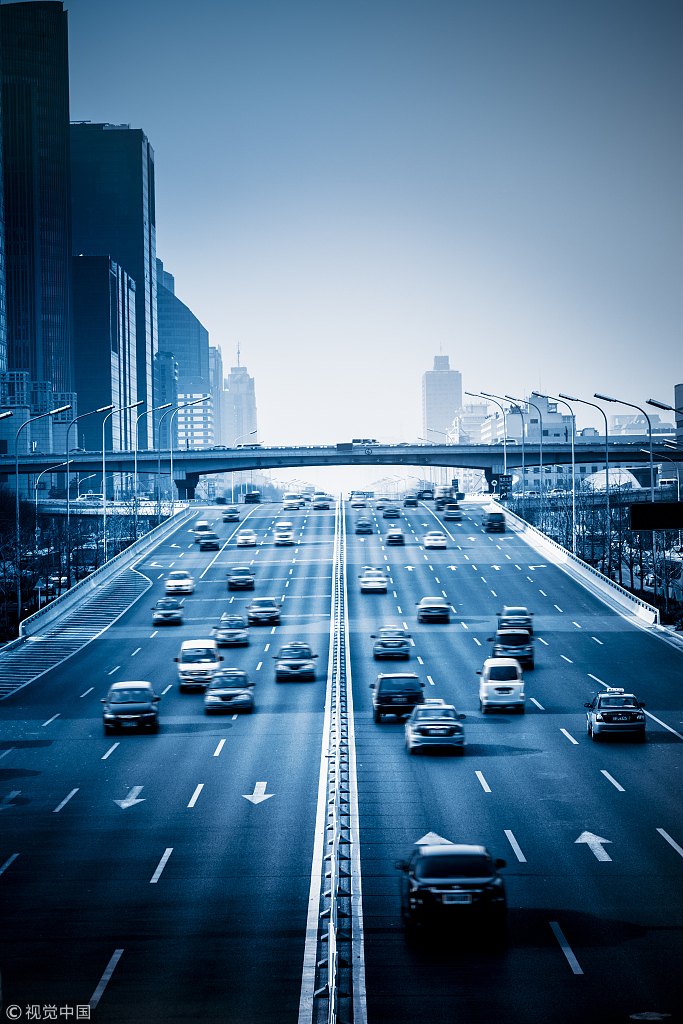
Overview of Traffic Sensor Technology
Traffic sensors refer to various devices installed on roads for real-time monitoring and collection of traffic data. Common traffic sensors include:
- Circular coil sensor: detects the presence and speed of the vehicle.
- Radar sensors: measure the speed and distance of vehicles.
- Video surveillance cameras: capture images for identifying vehicle types, counting, and analyzing behavior.
- Infrared sensor: detects the passage time and interval of vehicles.
- Smart checkpoint: records the time of vehicle entry and exit, assisting traffic management.
Data acquisition and processing
In order to effectively utilize traffic sensor data, a reliable data collection system must be established. The system includes:
- Data collection: Collect various traffic parameters such as vehicle quantity, speed, direction, etc. through sensors.
- Data transmission: The collected data is sent to the central processing unit through wired or wireless networks.
- Data preprocessing: cleaning, denoising, filling missing values, etc. to ensure data quality.
- Data storage: Utilizing database technology to store large amounts of data for easy analysis and use in later stages.
Optimization of Traffic Flow Model
A traffic flow model is a mathematical model used to describe and predict the dynamic characteristics of traffic flow. Optimizing traffic flow models using sensor data mainly involves the following aspects:
- Model selection: Choose the appropriate model type based on the characteristics of traffic data, such as macro model, micro model, or mixed model.
- Parameter calibration: Use sensor data to calibrate model parameters to make them more closely aligned with actual traffic conditions.
- Dynamic adjustment: Adjust the model prediction results dynamically based on real-time data to adapt to the constantly changing traffic environment.
Specific application cases
1. Traffic signal optimization
- By analyzing real-time traffic flow data and dynamically adjusting signal timing schemes, traffic delays can be reduced.
- Implement a green wave strategy, which coordinates signal light cycles on main roads to enable vehicles to pass through multiple intersections continuously without stopping.
2. Accident prediction and prevention
- Utilize sensor data to identify anomalies in traffic patterns, such as sudden deceleration, emergency braking, etc., and promptly issue warning signals.
- Through historical data analysis, identify high-risk accident areas and take targeted measures to reduce accident rates.
3. Intelligent path planning
- Based on real-time traffic conditions, recommend the optimal driving route for drivers and avoid congested roads.
- In the urban planning stage, use historical traffic data to predict future traffic flow and plan road layout reasonably.
Technical Support and Development Trends
With the development of big data technology and artificial intelligence, the application scope of traffic sensor data is constantly expanding. For example:
- Big data analysis platform: Utilizing cloud computing technology to process massive traffic data and quickly extract useful information.
- Machine learning algorithms: automatically recognize traffic patterns through training models to improve prediction accuracy.
- Internet of Things (IoT) technology: Connecting various sensors to form a massive IoT system, enabling data sharing and collaboration.
Optimizing traffic flow models using traffic sensor data is an important means of improving urban traffic efficiency. Real time monitoring, data processing, and analysis can provide scientific basis for traffic management, reduce congestion, and improve road utilization. With the continuous development of technology, we have reason to believe that future urban transportation will be more intelligent and efficient.