Deep Application and Improvement of Video Detection Technology in Intelligent Transportation
In the era of rapid technological development, intelligent transportation systems have become an important part of urban modernization. Among them, video detection technology, with its unique advantages, plays a crucial role in the field of intelligent transportation. It mimics the human eye’s visual mechanism and integrates advanced image processing algorithms to accurately extract the motion trajectories, spatial positions, and behavioral characteristics of traffic targets from complex video streams, providing key data support for traffic management and helping to achieve an efficient, safe, and intelligent traffic environment.
I. Core Principles and Evolution of Video Detection Technology
The core principle of video detection technology is to imitate the human visual perception process. Through a series of complex and sophisticated technical means, it deeply mines and analyzes the traffic information in videos. Its core technologies cover several key areas:
1. Object Detection and Tracking
The YOLO series of algorithms based on deep learning (such as YOLOv8), being outstanding in the field of object detection, adopt a one – stage detection framework. This framework breaks the traditional two – stage processing model of detection algorithms, greatly improving the detection speed. It can real – time locate targets such as vehicles and pedestrians in videos within a short time. In the complex urban traffic scene, a large number of image frames may need to be processed per second. Thanks to its efficient network structure and fast computing power, YOLOv8 can quickly identify the positions and categories of various targets, providing basic data for subsequent traffic analysis.
The DeepSORT algorithm focuses on solving the problem of multi – object cross – frame tracking. In actual traffic scenes, vehicles and pedestrians are constantly moving, and there may be situations such as occlusion and deformation of targets between different frames, which pose great challenges to tracking. The DeepSORT algorithm uses the Kalman filter technology to predict and update the motion state of targets. Combined with re – identification features, even if the appearance of the target changes in different frames, it can accurately determine its identity, ensuring the continuity of the target trajectory. For example, on a street with heavy traffic flow, vehicles frequently occlude and crisscross. The DeepSORT algorithm can stably track each vehicle, providing accurate trajectory data for traffic flow statistics and behavior analysis.
Traditional methods such as the optical flow method and stereo vision technology also play important roles in complex scenes. The optical flow method calculates the motion vectors of pixels by analyzing the changes in pixel intensity in images, thereby obtaining the motion information of targets. In some special scenarios, such as at night or in low – light environments, the optical flow method can assist deep – learning algorithms to more accurately identify the motion trajectories of targets. Stereo vision technology uses the parallax information obtained from binocular cameras to estimate the depth of targets and achieve 3D positioning of targets. In complex traffic scenes, it helps to more accurately determine the position and distance of targets.
2. Scene Understanding and Behavior Analysis
Semantic segmentation technology (such as U – Net) plays a key role in video detection. It can perform pixel – level annotation of traffic elements such as roads, lane lines, and traffic lights. Through this fine – grained annotation, the system can clearly distinguish different traffic scene elements. Combined with spatio – temporal context information, the system can determine whether vehicles have committed violations such as crossing the line and driving in the opposite direction. At a crossroads, by processing the video image with semantic segmentation technology, the system can accurately identify the lane lines and the positions of vehicles. Then, according to the motion trajectories of vehicles over a period of time, it can determine whether they violate traffic rules.
Dynamic event detection algorithms can identify sudden situations such as accident stops, traffic jams, and pedestrians crossing the road by deeply analyzing sequence images. It not only pays attention to the information in a single image but also comprehensively considers the changes in multiple consecutive images. Once an abnormal event is detected, the system will immediately trigger a real – time warning and notify the traffic management department to take timely measures. For example, when an accident stop is detected on a certain road section, the system will quickly issue an alarm and send the accident location and relevant image information to the traffic police department, so as to promptly divert traffic and reduce the impact of the accident on traffic.
3. Multimodal Data Fusion
With the continuous development of technology, the fusion of ultra – high – definition video (4K/8K) with millimeter – wave radar and LiDAR data has become an important means to improve the performance of video detection technology. Ultra – high – definition video provides rich visual details and can clearly present various elements in the traffic scene. Millimeter – wave radar and LiDAR have high – precision distance measurement capabilities, which can make up for the deficiency of video detection in distance perception. By fusing these different types of data, the detection range and accuracy can be expanded. On the highway, ultra – high – definition video can clearly capture the appearance and driving status of vehicles in the distance, while millimeter – wave radar and LiDAR can accurately measure the distance and speed of vehicles. The combination of the two can provide a more comprehensive and accurate understanding of the traffic situation.
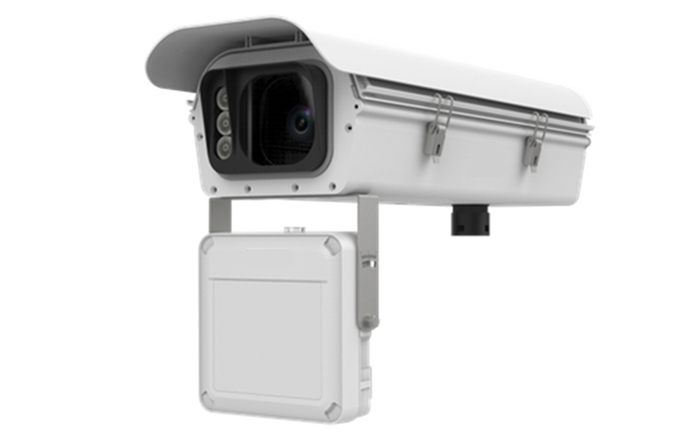
II. Deep Application Scenarios and Industry Empowerment
With its powerful functions, video detection technology has been widely integrated into various aspects of traffic management, bringing significant efficiency improvements and safety guarantees to the entire industry.
1. Intelligent Traffic Violation Evidence Collection
The electronic police system, an important tool for traffic violation evidence collection, makes full use of the license plate recognition (VLPR) and behavior analysis functions in video detection technology. It can automatically capture up to 12 types of traffic violations, such as running red lights, crossing the yellow line, and not driving in the designated lane, with an identification rate of over 99%. At various intersections in the city, the electronic police system operates 24 hours a day. It captures the driving images of vehicles through high – definition cameras, uses advanced algorithms to recognize license plates, and analyzes the driving trajectories and behaviors of vehicles at the same time. Once a traffic violation is detected, it will be immediately captured and the relevant information will be recorded, providing strong evidence for traffic law enforcement.
Drone – based law enforcement brings new perspectives and flexibility to traffic management. Drones equipped with high – definition cameras can easily reach areas that are difficult to cover by traditional devices, such as emergency lanes and tunnels, which are blind spots in traffic management. After a certain city piloted drone – based law enforcement, the incidence of violations such as emergency lane occupation and lane – changing in tunnels decreased by 40%. Drones can conduct real – time monitoring of specific areas from high altitudes. Once a traffic violation is detected, they can capture and record it in a timely manner, effectively curbing the occurrence of such violations.
2. Traffic State Perception and Optimization
Traffic flow monitoring is an important part of traffic management. The vehicle counting algorithm based on deep learning can real – time count key information such as the traffic flow density and speed at intersections. By analyzing video images, the algorithm can accurately identify vehicles and calculate their numbers and speeds. These data provide strong data support for signal timing optimization. According to the real – time traffic flow situation, the traffic management department can adjust the duration of traffic lights to make the traffic flow smoother. At a busy intersection, by monitoring the traffic flow density and speed in real – time and appropriately extending the green light time for the direction with heavy traffic, traffic congestion can be effectively alleviated.
Traffic congestion warning is also an important application of video detection technology in traffic management. By combining historical data with real – time video information, the system can predict the spread trend of traffic congestion and generate reasonable detour plans. After a certain highway section applied this technology, the accident response time was shortened by 30%. When the system detects signs of traffic congestion on a certain road section, it will quickly analyze historical data and real – time traffic conditions, predict the possible spread range and time of the congestion, and at the same time provide detour suggestions for drivers to guide vehicles around the congested section and improve the road traffic efficiency.
3. Safety Prevention and Control and Emergency Response
In the rapid handling of accidents, drones play an important role. They can reach the accident scene within 5 minutes and assist in rescue through 3D modeling and thermal imaging technology. In a certain highway accident case, the drone used 3D modeling technology to quickly model the accident scene, providing rescue workers with detailed information about the on – site terrain and vehicle positions. The thermal imaging technology helped rescue workers quickly find trapped people, greatly improving the rescue efficiency by 30%.
Driver behavior monitoring is also an important measure to ensure traffic safety. Based on facial recognition and eye – tracking technology, the system can real – time detect risks such as fatigue driving and distracted driving by the driver. Once abnormal behavior is detected, the system will immediately trigger an audible and visual alarm to remind the driver to pay attention to safety. Statistics show that the application of this technology has reduced the human – caused accident rate by 60%, effectively guaranteeing road traffic safety.
4. Intelligent Parking and Road Network Planning
In the field of intelligent parking, video detection technology shows great advantages. A single camera can monitor hundreds of parking spaces, replacing traditional high – cost geomagnetic sensors. By analyzing video images, the system can real – time obtain the status of parking spaces and achieve “what you see is what you get” vacancy guidance. In a large – scale parking lot, car owners can view the real – time vacancy information in the parking lot through a mobile phone APP and quickly find an empty parking space, improving the parking efficiency.
In road network planning, drone aerial photography combined with video analysis technology can generate high – precision 3D road network models. Planning departments can use these models to more intuitively understand the layout of urban roads and traffic flow conditions, thereby optimizing the road layout and improving the overall operation efficiency of urban traffic.
III. Technical Bottlenecks and Improvement Directions
Although video detection technology has made significant progress in the field of intelligent transportation, it still faces some challenges in practical applications and needs to be continuously improved and optimized.
1. Insufficient Adaptability to Complex Environments
In actual traffic scenes, adverse weather conditions such as rain, snow, fog, and low – light can seriously affect the accuracy of video detection. In rainy and snowy weather, raindrops and snowflakes can block targets, resulting in the loss of some target information. Foggy weather can make images blurry and reduce the 辨识度 of targets. In low – light environments, the contrast and brightness of images are insufficient, making it difficult to accurately identify targets. In addition, occlusion and perspective distortion can also lead to missed target detection. In traffic jams, vehicles often block each other, which poses great difficulties for target detection.
To address these issues, the Transformer architecture can be introduced. The Transformer has a powerful global feature extraction ability and can better handle the problem of target tracking in occluded scenes, improving the robustness of tracking. The use of multi – spectral imaging technology, which combines visible light and infrared light, can obtain more target information and enhance the image quality in adverse weather conditions. Combining with an adaptive denoising algorithm can effectively remove noise from images, enhance image clarity, and improve the accuracy of target detection.
2. Contradiction between Real – Time Performance and Computing Power
With the continuous improvement of video resolution, such as the wide application of 4K video, the requirement for video processing computing power is also increasing. However, due to limited hardware resources, edge devices often struggle to meet the needs of real – time processing of 4K video. In practical applications, if video data cannot be processed in a timely manner, it will lead to delays in detection results, affecting the timeliness and accuracy of traffic management.
To solve this contradiction, model lightweighting technology can be adopted. For example, lightweight networks such as MobileNet and ShuffleNet can be used to compress the number of parameters of YOLOv8, enabling it to run quickly on embedded devices. Through the edge – cloud collaboration method, preliminary detection work is completed at the camera end, and complex analysis tasks are uploaded to the cloud for processing. This can greatly reduce data transmission delays and improve the real – time performance of the system.
3. Weak Cross – Scene Generalization Ability
There are significant differences in road structures and vehicle types in different cities and regions. Models trained for specific scenes often struggle to adapt to these differences when applied to new areas, resulting in a decline in detection accuracy. In some cities, the roads are narrow and the traffic flow is heavy, while in other cities, the roads are wide and the vehicle types are diverse. The application effect of the same model in different cities may vary greatly.
To enhance the cross – scene generalization ability of models, self – supervised learning methods can be adopted. A large amount of unlabeled video data can be used to pre – train the model, allowing the model to learn more general features and thus improving its adaptability in different scenes. Federated learning is also an effective method. Traffic departments in multiple cities can share model parameters while protecting data privacy and jointly train to improve the generalization ability of the model, enabling it to better adapt to traffic scenes in different regions.